May 24, 2024
Interview with Danilo Bzdok, Scientific Co-Director, Research Programs and Academic Relations
Professor at McGill University, Danilo Bzdok, a renowned neuroscientist and specialist in predictive model learning, is Co-Scientific Director in charge of Research Programs and Academic Relations at IVADO. In this interview, he reveals the challenges and opportunities that have marked his career, and shares his vision for the future of interdisciplinary research.
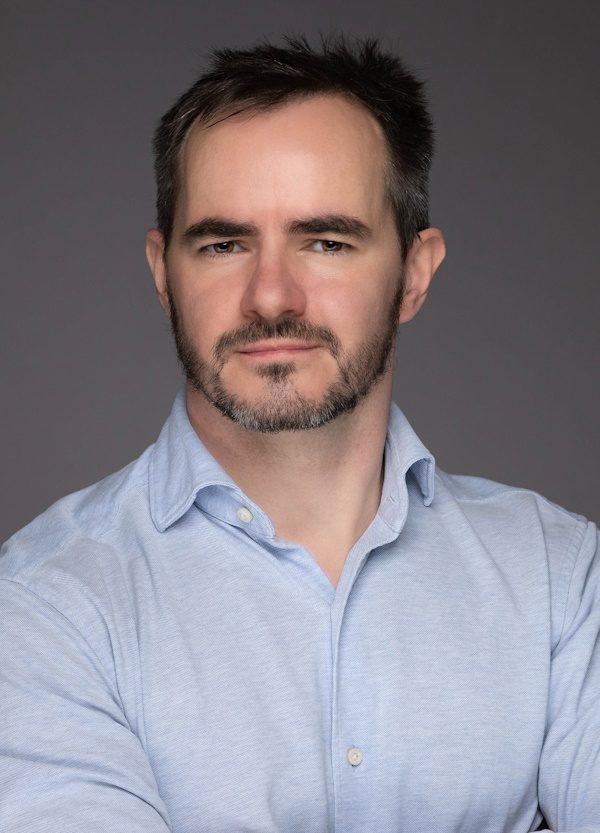
1- You are a researcher specializing in the neurosciences and neurobiology of human intelligence. How do you see the role of AI in the field of biomedical sciences and neurosciences?
Neuroscience and medicine are currently changing at a more rapid pace than probably ever before. Over the last 10 or so years, the neurosciences and biomedicine have entered the age of “big data”. There is a growing momentum towards data sharing, open access, and building “big data” repositories. For example, one of my team’s favorite datasets is the UK Biobank – a longitudinal population study dedicated to the genetic and environmental influence on mental disorders and other medical conditions. 500,000 enrolled volunteers underwent an extensive battery of clinical diagnostics from brain scans to bone density with a > 25 year follow-up.
On the other hand, the invention and application of statistical tools has historically always been dictated by changing contexts and domain questions. Data analysis practices and choices can and should change due to the progressively increasing granularity of digitized data on the brain and body. Targeted analysis and re-analysis of such national and international data collections may soon become the new normal in basic and clinical neuroscience. I therefore think that investigators have to extend their modeling instincts towards new flavors of data science tools – this is where AI comes into play.
2- How are recent advances in artificial intelligence contributing to our understanding of the brain and cognitive processes?
Large language models (LLMs) are a new asset class in the deep-learning landscape, they are currently fueling yet another wave of momentum in the AI ecosystems. The expansion and explosion of LLM architectures was fueled by (1) the invention of transformers, which tend to vary only slightly across recent LLMs, (2) availability of abundant, Internet-scale data sources, and (3) availability of compute power at scale.
From my vintage point, neuroscience investigators can leverage this emerging toolkit in a number of ways:
- LLM learning engines immediately appear appropriate not only for word sequences, but also for different kinds of biological sequences: the one-directional flow of genetic information from (1) nucleotide sequences in DNA to (2) base sequences in messenger RNA to (3) amino acid sequences in protein products. LLMs serve as a lever that now enables advanced in-silico models of the central dogma of biology (going from the DNA double helix to gene transcript expression to fully formed proteins), with a special potential in AI-based drug discovery.
- Neuroscience research critically hinges on accurate annotation for data elaboration, designing experiments, or interpretation of results. Hence, automatically derived semantic facets may be more successful in a variety of studies than traditional approaches that rely on human a priori intuition. Pipelining annotation generation could dramatically enhance our capabilities to scale complex manual protocols in different forms of the “data real estate”, such as electronic health records (eHR), voice recordings, movie scenes, or the biometric outputs captured by wearable devices.
- We can identify new classification and description systems about cognition and neurobiological entities in health and disease by using LLM-assisted annotations as a complementary approach to supplement legacy top-down and standard rule-based solutions by truly letting the data ‘‘speak for themselves.’’
- Hence, as one possible future scenario, emerging LLM technologies can spark advances toward a biologically grounded redefinition of major brain disease nosology, cutting across diagnostic boundaries in a new era of evidence-based medicine, rather than relying on the judgment of a few selected experts alone.
- Moreover, LLMs may provide an alternative toolkit that turns out to be valuable to audit and edit the human-conceived notions that neuroscience investigators act on to understand the brain. It is important to appreciate that, especially in classically narrow hypothesis-driven research, the entire research endeavor hinges on the pre-assumed validity of the cognitive and neural terms that were used to articulate the experimental research conditions.
In short, it is my conviction that neuroscientists need to be, both, more humble and more bold. More humble in being open to the possibility that we may find better explanatory theories of what is going on in neural brain circuits than the ideas that are being passed down from one generation of scientists to the next. More bold in embracing drastically data-driven analysis paradigms to mine, combine and make sense of massive data troves.
3- What role do you see IVADO playing in the AI research ecosystem, particularly in the neurosciences?
IVADO is the largest publicly funded AI endeavor in Canada. This consortium aims to promote, both, core AI and AI applications by consolidating efforts of stakeholders at multiple levels at University of Montreal, McGill University, HEC Montréal, Polytechnique Montréal, and University of Laval; with partnerships extending well beyond these institutions.
We entertain scientific research activities together with 300+ university professors and liaise the cross-sectoral agendas between 16 institutes and centers (academic members). The project is dedicated to ensuring that AI development and deployment are responsible and inclusive. Its primary mission is to transform scientific discoveries into practical applications, generating economic and social value; and also to develop and promote the use of artificial intelligence (AI) and data science across various sectors, by placing a premium on translating core research insights into the real-world impact that is beneficial to society as a whole. We aim to foster collaboration among academic researchers, industry partners, and government bodies to drive innovation and digital transformation. IVADO’s DNA directly aligns with Quebec’s and Canada’s strategic goals to become global leaders in ethical AI.
In terms of its possible impact on neuroscience, I believe that state-of-the-art AI may have a reconciliatory effect on what is currently a highly fragmented mosaic of research activity. The wide-ranging ballpark that is neuroscience touches various disciplines from physics to psychology. This wildly interdisciplinary field produces a myriad of rather separate experimental findings that can be overwhelming to integrate by human effort alone. Moreover, the breadth of the field often results in researchers working within a particular sub-community, focusing on narrowly specialized research areas, and potentially missing out on opportunities through potential cross-fertilization with other sub-disciplines. While separate neuroscience pockets have become increasingly idiosyncratic over the recent years and decades, with their own agendas, beliefs and experimental paradigms, convergence on emerging AI solutions may help reunite disparate neuroscience research activities.
As a contrarian, I am going to say that many pockets of the neurosciences do, today, still not possess the data resource that they would need to respond to the research questions they are asking in a satisfying way. Despite the “big-data” movement, many areas of neuroscience do not have the internet-scale kinds of datasets that fuel text and image analysis in the AI community. Neuroscientists should therefore unite in wondering what kinds of non-neuroscience information can be leveraged to make them AI-actionable? This ‘‘transfer learning’’ goal refers to a mode of data analytics that revolves around storing structured knowledge gained while solving one problem, for applying it to a different but related problem, in what may require community-wide efforts and data sharing. LLMs, and other transformer-carrying architectures, have shown beyond-expectations capability in transfer learning. Emerging, more powerful AI models, ported from general data to neuroscience-relevant data, can then serve as a platform to revisit and attack classic neuroscience problems from a fresh angle. In a next step, these community-specific AI models (foundation models) can then be used in conjunction to build bridges of investigation across different levels of biology (cells, neural networks, mesoscale, macroscale, environment, society, …) that were previously unthinkable. Such a research agenda could help overcome what appears to me to be neuroscience communities being siloed operating in their ‘own ‘bubble’.
Enabling researchers across diverse neuroscience domains to bootstrap common building blocks may also help boost comparability across studies and foster collaboration across teams, institutions, and geographies. Maybe human effort alone is not enough to formally coalesce the research products of some >100,000 neuroscientists on the planet… Such knowledge fragmentation is, in my subjective perception, maybe one of the biggest challenges of the scientific enterprise in the 21st century.
As a core aspiration for the intensely interdisciplinary neuroscience endeavor, recent AI technologies can help us overcome the divide between disparate neuroscience communities and can enable us to form integrative across-community model-fusion solutions that can amalgamate the knowledge mosaics. Perhaps scientists need to partner with AI to have a shot at understanding how the neural networks in their own head’s work.
4- What are your priorities as Scientific Co-Director to stimulate innovation in the priority areas set out in the R³AI project?
As my main priority as a member of the IVADO leadership, I want to help create a unique research environment in Quebec that enables investigators to carry out exceptionally ambitious and disruptive AI projects, with a recognized international footprint.