April 9, 2025
IVADO Unveils Seven New Research Projects Funded Through its 2024-25 Exploratory Projects Program
Montreal, April 9, 2025 – IVADO is pleased to unveil seven new research projects funded through its Exploratory Projects program. These initiatives add to the three award-winning projects announced last November.
Launched last year, this program supports small to medium-sized research projects that are innovative, bold and ideally cross-sectoral or interdisciplinary, enabling the exploration of new ideas related to the R3AI initiative. In particular, the program aims to support the development of projects during their exploratory phase, notably by funding graduate students and postdoc researchers working on these projects. You can visit the Exploratory Projects program page for more details.
Seven New Funded Projects
Simulations to Solve Societal-Scale Manipulation
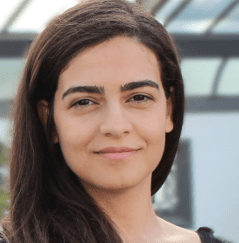
Principal Investigator: Reihaneh Rabbany, School of Computer Science (McGill)
Co-Investigator: Jean-François Godbout (Université de Montréal)
Funding awarded: $100,000
This project will develop a social system simulator to study and defend against AI-driven manipulation in online communities. The simulator will use Large Language Models to create realistic social environments and agent behaviors. This will allow for the testing of various defenses against manipulation tactics, enhancing our understanding of AI threats and informing policy decisions.
The Psychological Landscape of Large Language Models: A Study of Robustness, Scaling, and Fine-Tuning of Personality Traits for Responsible AI
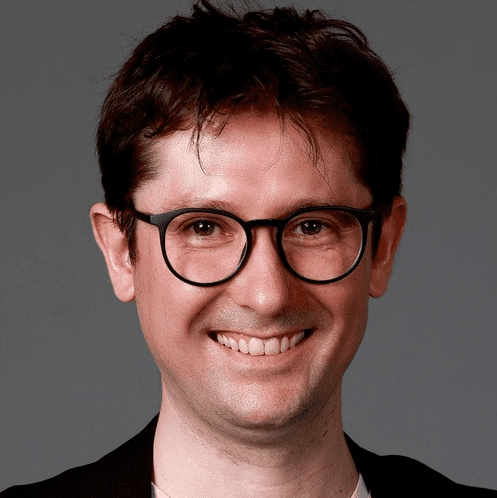
Principal Investigator: Guillaume Dumas, Department of Psychiatry and Addiction (Université de Montréal)
Co-Investigators: Irina Rish (Université de Montréal), Tommaso Tosato (Université de Montréal)
Funding awarded: $100,000
Our project studies how large language models (like ChatGPT) display various socio-emotional responses, and evaluates their safety for sensitive applications like mental health support and education. We’re developing innovative tests to measure whether these response patterns remain consistent or fluctuate unexpectedly during extended interactions. Our team is also creating techniques to fine-tune these AI behaviors, making them more reliable and appropriate when interacting with people in sensitive settings.
Exploratory Project: Mapping and LLM-Alignment of Human Cognitive Dimensions Using Psychedelics Research and Foundation Models
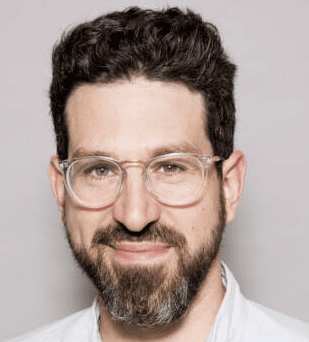
Principal Investigator: Guillaume Lajoie, Department of Mathematics and Statistics (Université de Montréal)
Co-Investigators: Danilo Bzdok (McGill); Thomas Ray, Paul Wren, Nicholas Cammarata (Mindstate)
Funding awarded: $100,000
This project harnesses pharmacological and phenomenological data on psychedelics to develop machine learning models that simulate the molecular mechanisms underlying human consciousness. In the short term, these models could accelerate the development of targeted psychedelic therapies that act on specific dimensions of consciousness. In the long term, the research aims to align large language models (LLMs) with human cognitive processes—enhancing their reasoning and interactions, and unlocking new possibilities for clinical applications in mental health and beyond.
Causal Climate Emulator
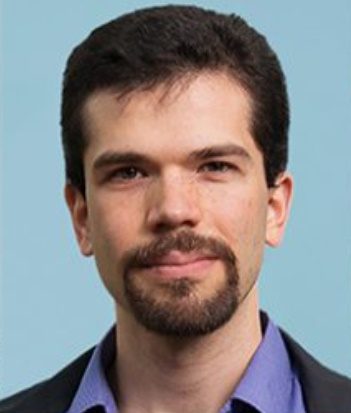
Principal Investigator: David Rolnick, School of Computer Science (McGill)
Co-Investigator: Peer Nowack (Karlsruhe Institute of Technology)
Funding awarded: $100,000
As climate change brings increased storms, fires, droughts, and temperature extremes, accurate climate modeling is increasingly crucial. We are using AI tools to help speed up climate models, which can otherwise be too slow to run at high precision. Specifically, we are designing AI-based causal emulators which use data to learn cause-and-effect relationships in the climate, then use those relationships to rapidly predict what will happen next.
Accelerating Scientific Discoveries of the Cosmos with Advanced AI in Radio Astronomy
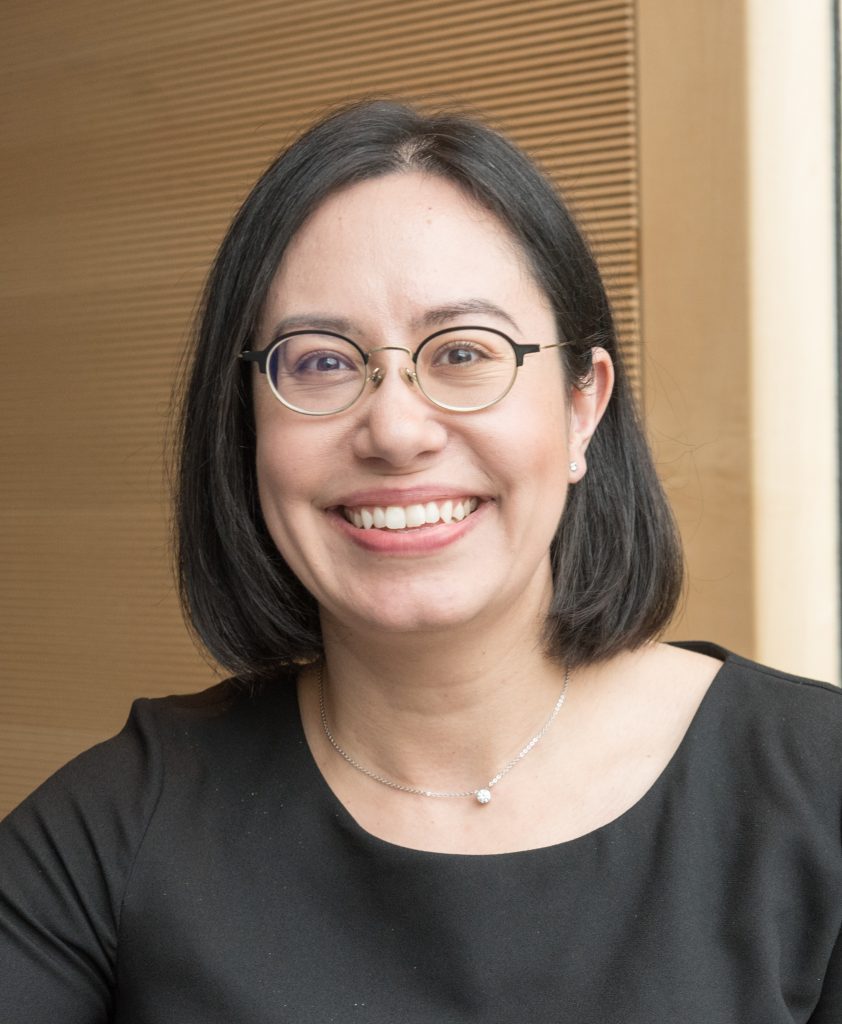
Principal Investigator: Julie Hlavacek-Larrondo, Department of Physics (Université de Montréal)
Co-Investigators: Sarath Chandar (Polytechnique), Kristine Spekkens (Queen’s), Siamak Ravanbakhsh (McGill), Adrian Liu (McGill), Laurence Perreault-Levasseur (Université de Montréal)
Funding awarded: $97,000
One of the largest scientific endeavours in history, the Square Kilometre Array Observatory (SKAO), is set to deliver images so groundbreaking they could transform our understanding of black holes, dark matter and energy—and even reveal the first signs of life beyond Earth. Yet a major challenge remains: each image is blurred by the instrument itself, and correcting this distortion demands immense computational power. This project aims to revolutionize astrophysics by developing a new class of neural networks for radio astronomy, leveraging cutting-edge AI to automate and accelerate image reconstruction, and ushering in a new era of cosmic discovery.
New Method for Precision Medicine With Count Outcomes That Addresses Informative Censoring: Improving the Quality of Care for Individuals With Multiple Sclerosis
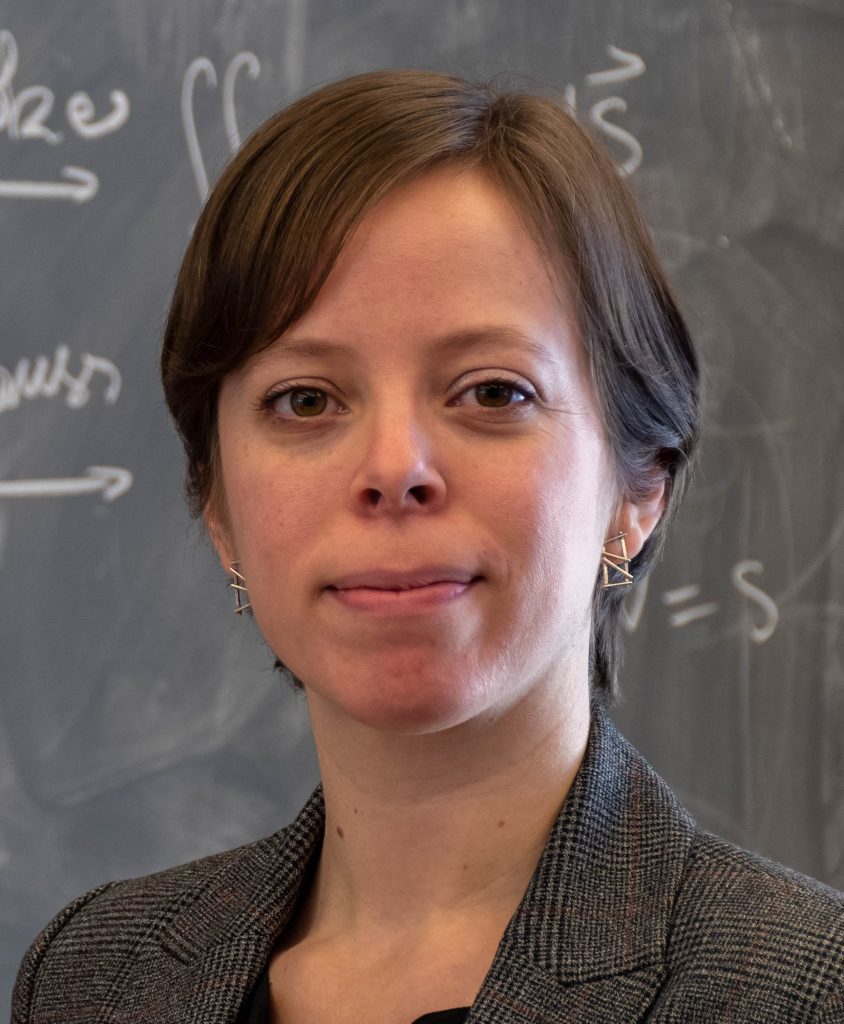
Principal Investigator: Janie Coulombe, Department of Mathematics and Statistics (Université de Montréal)
Co-Investigators: Miceline Mésidor (INRS), Mireille Schnitzer (Université de Montréal), Christel Renoux (McGill)
Funding awarded: $57,500
The goal of this project is to develop a robust and efficient new statistical approach to derive optimal treatment rules for individuals living with multiple sclerosis. These rules will be designed to identify the best treatment for a given patient based on their personal characteristics, with the aim of reducing relapse rates. The proposed method will account for various data complexities that can affect inference, including informative censoring due to different causes such as death.
Computational Efficiency Through Learning Perceptual Heuristics in Biological and Artificial Neural Networks
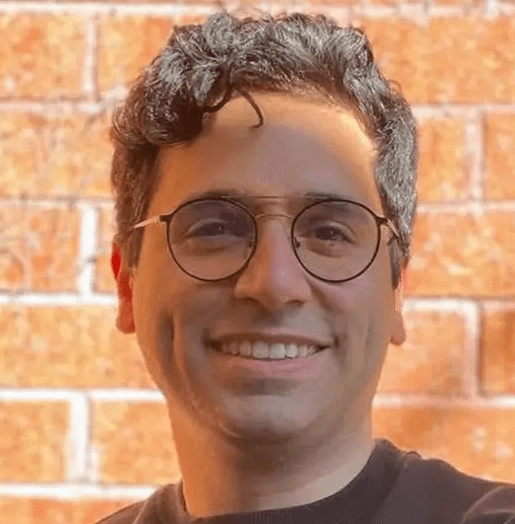
Principal Investigator: Shahab Bakhtiari, Department of Psychology (Université de Montréal)
Co-Investigator: Christopher Pack (McGill)
Funding awarded: $99,800
The human brain learns visual tasks using mental shortcuts (or perceptual heuristics) that consume less energy than the intensive optimization methods used by artificial intelligence (AI) systems. Adopting these strategies may explain why the brain can continuously learn using the energy of a light bulb while AI training can consume as much electricity as hundreds of households. This project aims to understand the neural mechanisms behind these efficient learning shortcuts to develop more energy-efficient AI systems.
Three Award-Winning Projects Unveiled Last November
Large-Scale Power Grids: Transition to Net Zero
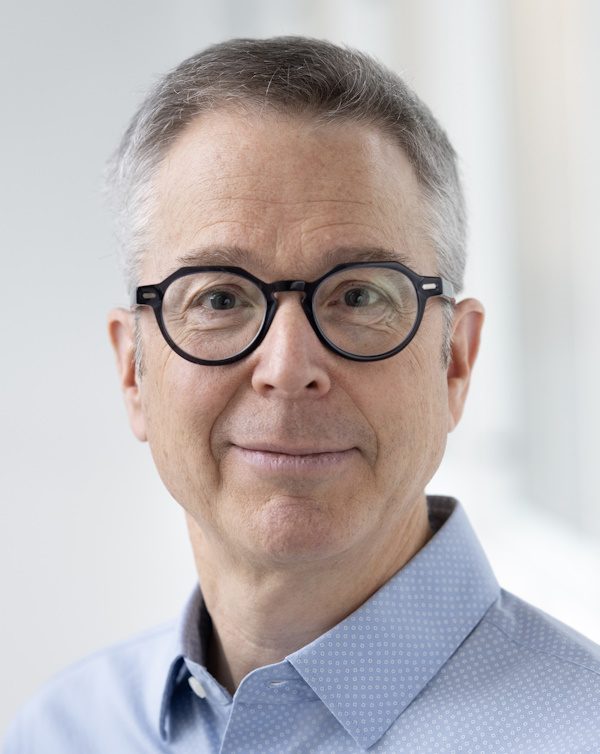
Principal Investigator: Michel Denault, Department of Decision Sciences (HEC Montréal)
Co-Investigators: Maryam Daryalal (HEC), Pierre-Olivier Pineau (HEC)
Funding awarded: $100,000
The transition to carbon-neutral energy systems presents new challenges, particularly due to the rise of renewable energy sources and their unpredictability. To address this, Professor Denault and his team are exploring the use of reinforcement learning to better plan the expansion of the electrical grid. They are also working to improve stochastic programming with neural networks to optimize the management of this complex network.
Harnessing Root Architecture and Anatomy Using Artificial Intelligence to Improve Soil Health and Resource Acquisition in a Changing Climate
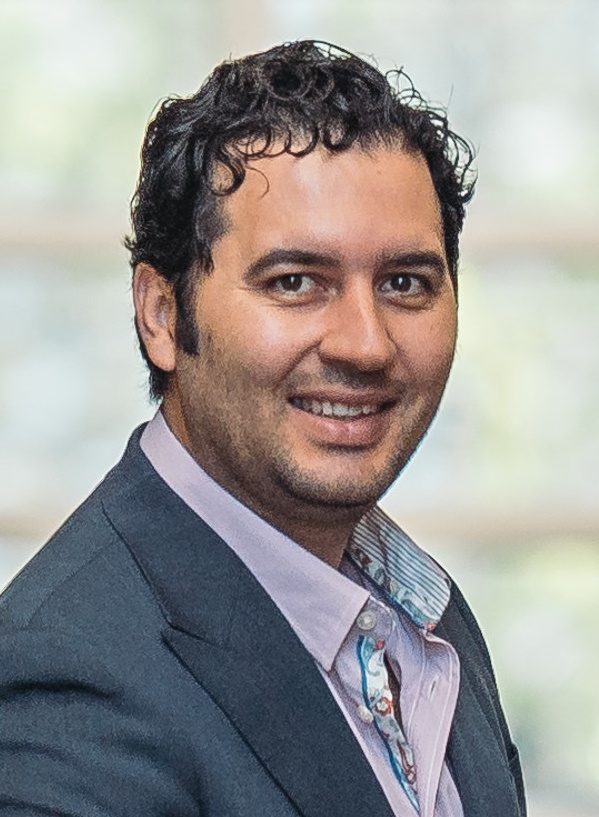
Principal Investigator: Davoud Torkamaneh, Department of Phytology (Université Laval)
Co-Investigator: Shangpeng Sun (McGill)
Funding awarded: $100,000
Plant roots, although crucial, remain under-studied despite their impact on climate resilience, CO₂ absorption, and mineral acquisition. Professor Torkamaneh’s project aims to create an AI model to classify root systems using 3D images and convolutional neural networks. This initiative will lead to the creation of the first global database dedicated to roots, with tangible benefits for agriculture, including the development of soybean varieties better suited to the Canadian climate.
Accuracy Quantification and Control in Scientific Machine Learning
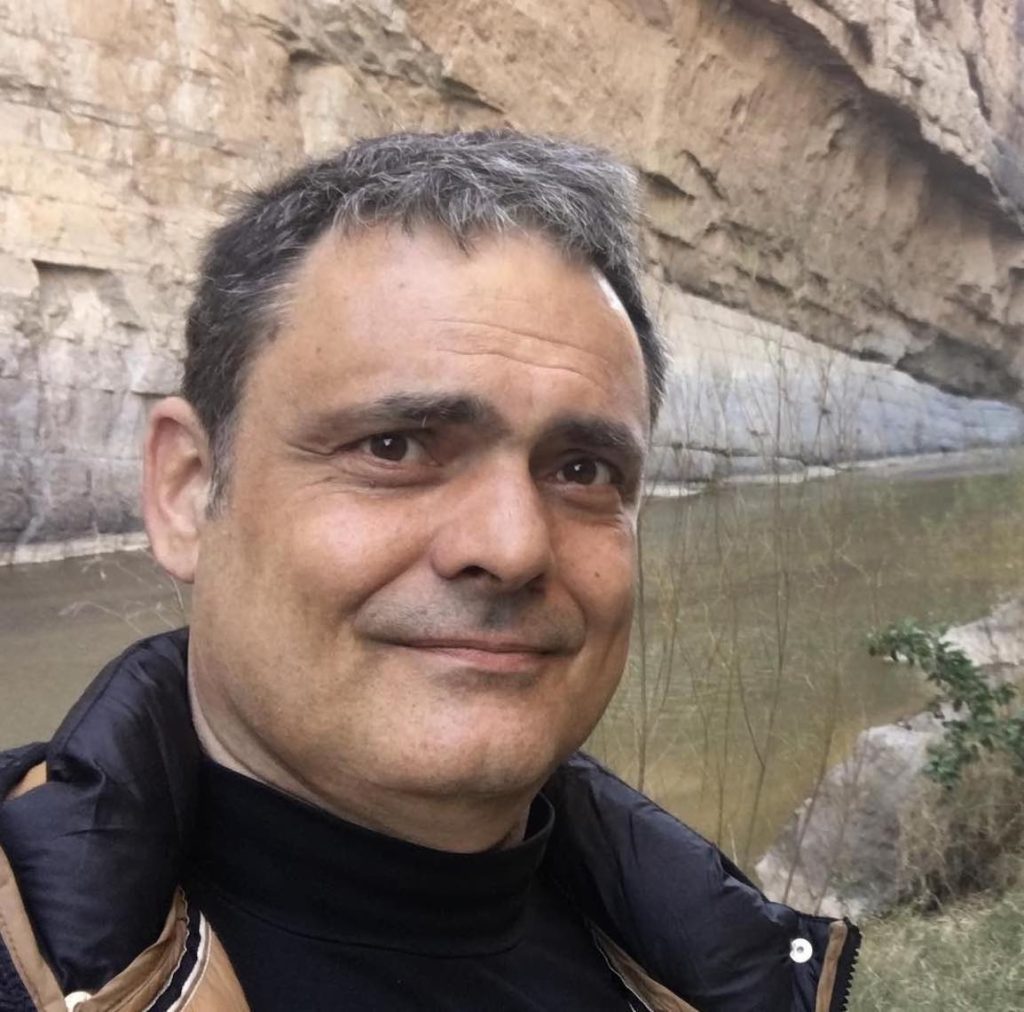
Principal Investigator: Serge Prudhomme, Department of Mathematics and Industrial Engineering (Polytechnique Montréal)
Co-Investigator: Simone Brugiapaglia (Concordia)
Funding awarded: $100,000
The development of new approaches in scientific computing based on machine learning presents challenges related to their interpretability and performance compared to traditional methods. This project aims to better equip machine learning practitioners for problem formulation, architecture design, sampling strategies, and hyperparameter tuning. The goal is to quantify and control the errors in deep learning approximations, with impacts in fields such as aerospace, mechanical engineering, and network science.